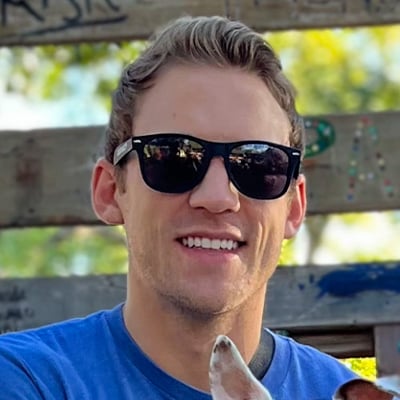
Michael Wall
Hi, I’m Michael Wall, a post-doc in the Hufnagel group at Johns Hopkins University, and I work in the artificial intelligence for material design laboratory. This lab is designed for collecting large data sets from multiple samples to train Machine Learning models for data analysis, and we have a team of people that have put this lab together. In the lab, I work on methods for high-throughput characterization of microstructure on high entropy alloys and ceramics using X-ray Diffraction and X-ray Fluorescence. We want to characterize these alloys and ceramics in a fast way which is difficult to do using conventional methods of diffraction and fluorescence, so we have designed and constructed a new instrument for high-energy transmission diffraction and integrated it into our artificial intelligence lab to look at bulk microstructure with minimal sample preparation. This new instrument combines a high-brightness, high-energy x-ray beam with a low-noise x-ray detector, and it also has robotic sample handling to allow for fast and easy cycling of samples which allows us to collect hundreds of diffraction and fluorescence data points in less than an hour. With the data that is collected in our lab, we stream it to a cloud server known as OpenMSI where other collaborators can access and process our data in real time. For example: We are currently working with people from Northwestern University to use our XRD data to train ML models for identifying phases of material. Overall, this combination of research enables autonomous data collection and analysis for high-throughput characterization of microstructure and chemical composition of these high entropy alloys and ceramics.