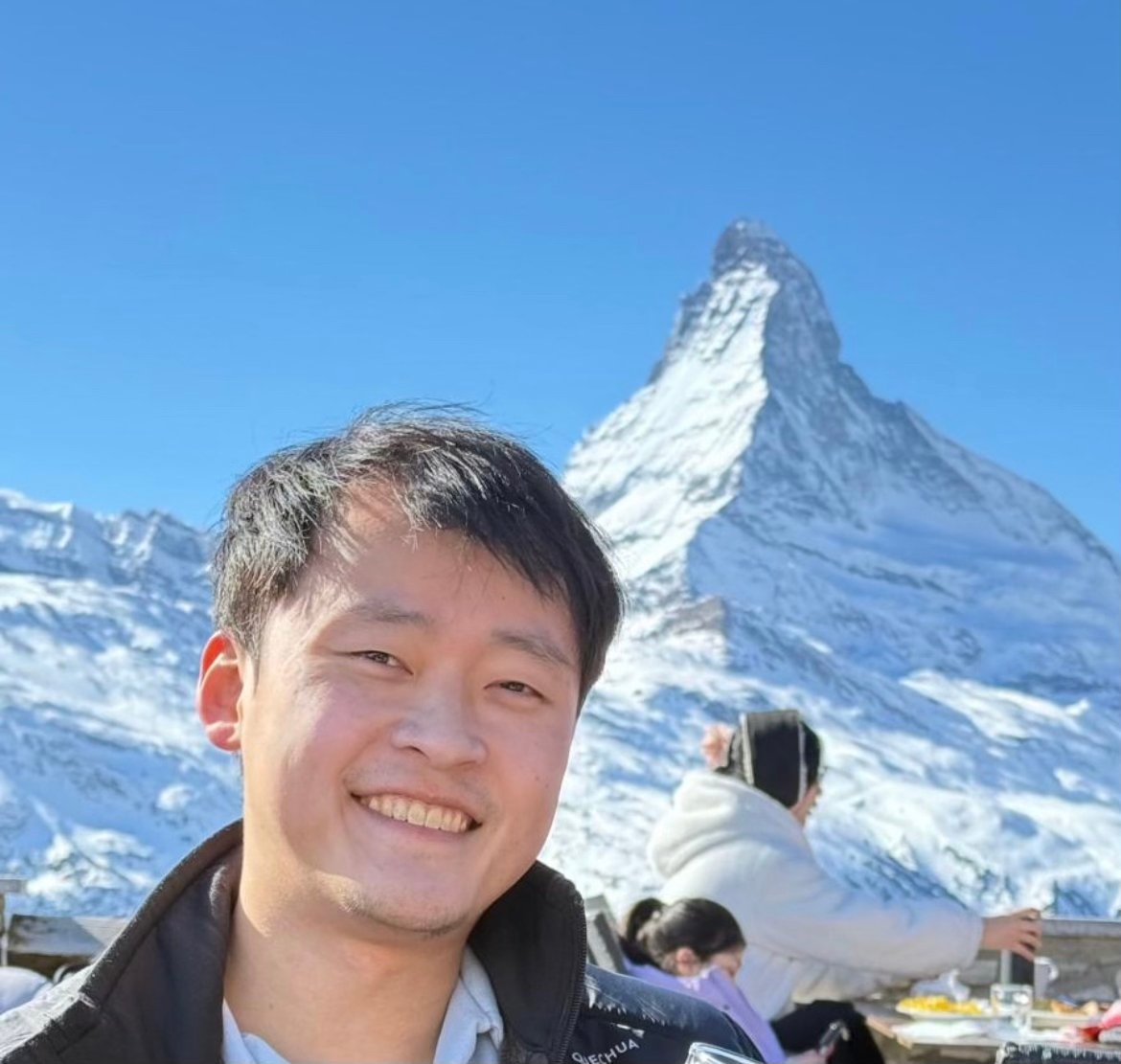
Harrison Park
Hello, my name is Harrison Park, and I work at the Artificial Intelligence for Materials Design Laboratory at Johns Hopkins University, also known as AIMD. Our lab is part of a broader initiative by the Hopkins Extreme Materials Institute to automate materials science research using AI-driven robotics and computational methods. Our primary goal is to develop a fully autonomous laboratory that can conduct experiments, analyze data, and make decisions continuously and without human intervention. Essentially, we’re aiming to replace manual processes with robotic systems that can characterize microstructure and mechanical behavior using advanced techniques such as rapid hardness testing or high-speed impact testing.
Currently, I am developing a new subsystem at AIMD for analyzing thermal properties. In certain applications, materials need to tolerate extreme environments, such as temperatures above 2000°C, without degrading. To predict how materials will perform under such conditions, we need to understand how properties such as thermal diffusivity, heat capacity, and thermal conductivity change in extreme environments. The Laser Flash Analysis method, which is a method of looking at how a laser pulse moves material, is commonly used for this purpose, but traditional high-temperature setups can take hours and involve separate measurements. My work involves reengineering the process to conduct experiments in fractions of a second and eliminate the need for prior measurements. By changing the traditional Laser Flash workflow, we can perform initial calculations without needing certain variables and then inverse-estimate the missing information after the experiment. This approach allows the system to operate autonomously, making it more compatible with a high-throughput lab.
The data we generate will eventually train machine learning algorithms that can help us make further decisions on what kind of materials research we wish to do at AIMD. While our goal is to remove manual intervention from the research process, there’s still a significant role for researchers in designing how these AI-driven subsystems perform measurements, analyses, and make decisions, and I hope to continue doing meaningful work in this sense.